


We train iGPT-S, iGPT-M, and iGPT-L, transformers containing 76M, 455M, and 1.4B parameters respectively, on ImageNet. In new domains, where there isn’t much knowledge to hand code, scaling compute seems an appropriate technique to test. However, in showing that an unsupervised transformer model is competitive with the best unsupervised convolutional nets, we provide evidence that it is possible to trade off hand coded domain knowledge for compute. Indeed, contrastive methods are still the most computationally efficient methods for producing high quality features from images. We deliberately chose to forgo hand coding any image specific knowledge in the form of convolutions or techniques like relative attention, sparse attention, and 2-D position embeddings.Īs a consequence of its generality, our method requires significantly more compute to achieve competitive performance in the unsupervised setting. Our work tests the power of this generality by directly applying the architecture used to train GPT-2 on natural language to image generation. Generative sequence modeling is a universal unsupervised learning algorithm: since all data types can be represented as sequences of bytes, a transformer can be directly applied to any data type without additional engineering. Then, through optimizing GPT-2 for generative capabilities, we achieve top-level classification performance in many settings, providing further evidence for analysis by synthesis. In our work, we first show that better generative models achieve stronger classification performance. Many early generative models were motivated by this idea, and more recently, BigBiGAN was an example which produced encouraging samples and features. Once it learns to do so, an idea known as “Analysis by Synthesis” suggests that the model will also know about object categories. In contrast, sequences of pixels do not clearly contain labels for the images they belong to.Įven without this explicit supervision, there is still a reason why GPT-2 on images might work: a sufficiently large transformer trained on next pixel prediction might eventually learn to generate diverse samples with clearly recognizable objects. One possible reason for this success is that instances of downstream language tasks appear naturally in text: questions are often followed by answers (which could help with question-answering) and passages are often followed by summaries (which could help with summarization). In language, unsupervised learning algorithms that rely on word prediction (like GPT-2 and BERT) have been extremely successful, achieving top performance on a wide array of language tasks. Logistic regression on learned features (linear probe) As further proof, features from the model achieve state-of-the-art performance on a number of classification datasets and near state-of-the-art unsupervised accuracy on ImageNet. This is evidenced by the diverse range of coherent image samples it generates, even without the guidance of human provided labels. When we train GPT-2 on images unrolled into long sequences of pixels, which we call iGPT, we find that the model appears to understand 2-D image characteristics such as object appearance and category. Transformer models like BERT and GPT-2 are domain agnostic, meaning that they can be directly applied to 1-D sequences of any form. Our work aims to understand and bridge this gap. However, the same broad class of models has not been successful in producing strong features for image classification.
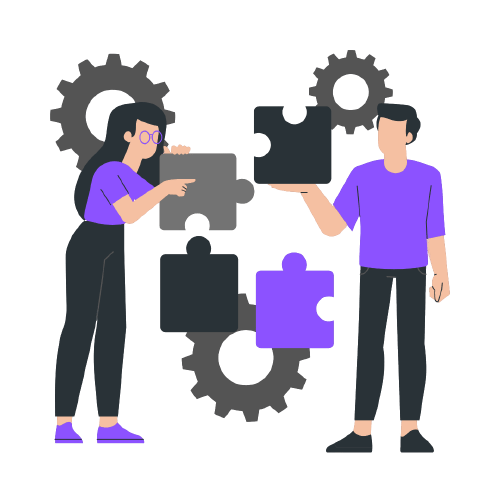
Recently, it has seen incredible success in language, as transformer models like BERT, GPT-2, RoBERTa, T5, and other variants have achieved top performance on a wide array of language tasks. Unsupervised and self-supervised learning, or learning without human-labeled data, is a longstanding challenge of machine learning.
